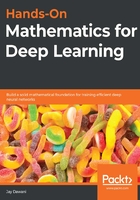
上QQ阅读APP看书,第一时间看更新
Chain rule
Let's take an arbitrary function f that takes variables x and y as input, and there is some change in either variable so that . Using this, we can find the change in f using the following:

This leads us to the following equation:

Then, by taking the limit of the function as , we can derive the chain rule for partial derivatives.
We express this as follows:

We now divide this equation by an additional small quantity (t) on which x and y are dependent, to find the gradient along . The preceding equation then becomes this one:

The differentiation rules that we came across earlier still apply here and can be extended to the multivariable case.